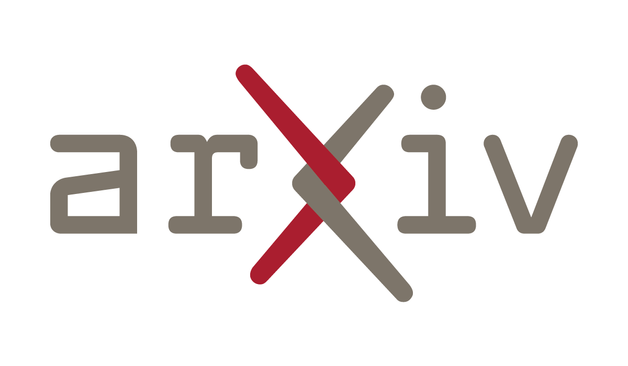
arXiv.orgPiccolo: Large-Scale Graph Processing with Fine-Grained In-Memory Scatter-GatheGraph processing requires irregular, fine-grained random access patterns incompatible with contemporary off-chip memory architecture, leading to inefficient data access. This inefficiency makes graph processing an extremely memory-bound application. Because of this, existing graph processing accelerators typically employ a graph tiling-based or processing-in-memory (PIM) approach to relieve the memory bottleneck. In the tiling-based approach, a graph is split into chunks that fit within the on-chip cache to maximize data reuse. In the PIM approach, arithmetic units are placed within memory to perform operations such as reduction or atomic addition. However, both approaches have several limitations, especially when implemented on current memory standards (i.e., DDR). Because the access granularity provided by DDR is much larger than that of the graph vertex property data, much of the bandwidth and cache capacity are wasted. PIM is meant to alleviate such issues, but it is difficult to use in conjunction with the tiling-based approach, resulting in a significant disadvantage. Furthermore, placing arithmetic units inside a memory chip is expensive, thereby supporting multiple types of operation is thought to be impractical. To address the above limitations, we present Piccolo, an end-to-end efficient graph processing accelerator with fine-grained in-memory random scatter-gather. Instead of placing expensive arithmetic units in off-chip memory, Piccolo focuses on reducing the off-chip traffic with non-arithmetic function-in-memory of random scatter-gather. To fully benefit from in-memory scatter-gather, Piccolo redesigns the cache and MHA of the accelerator such that it can enjoy both the advantage of tiling and in-memory operations. Piccolo achieves a maximum speedup of 3.28$\times$ and a geometric mean speedup of 1.62$\times$ across various and extensive benchmarks.